写的:

Senior Director, Head of Biosciences Renal, 澳门第一赌城在线娱乐 R&D、澳门在线赌城娱乐
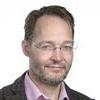
Executive Director, Data Sciences and Quantitative Biology, Discovery Sciences, R&D、澳门在线赌城娱乐
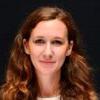
benevolentai首席产品经理
数据科学 and artificial intelligence (AI) have the potential to transform the way we discover and develop medicines. We know that selecting the right target with a strong link to the drivers of disease remains the most important decision we make in the drug discovery process, 数据科学和人工智能在这里产生了积极的影响.
在联合多学科团队中并肩工作, with leading expertise in drug discovery and disease understanding from AstraZeneca, 以及来自BenevolentAI的机器学习模型和人工智能, we are evolving drug discovery for some of the world’s most complex biological challenges. 澳门第一赌城在线娱乐一起采取了一种创新的, 数据驱动的目标发现方法, 使用BenevolentAI强大的AI模型和工具, to provide AstraZeneca scientists with new insights into disease biology in order to select novel targets that specifically treat the cause of disease.
We have access to more data than ever before but the value of this data can only be realised if we are able to analyse, 解释并应用它. We’ve had success in utilising human OMICs data to identify new targets but we wanted to take it to the next level. Data has historically been analysed in silos and we knew that the ability to better integrate datasets via a knowledge graph had the potential to be game changing.
Our collaboration focuses on two diseases with significant unmet need: chronic kidney disease (CKD) and idiopathic pulmonary fibrosis (IPF). These are also two complex multifactorial diseases where drug discovery is particularly challenging.
We started out by building disease-specific knowledge graphs for IPF and CKD. A knowledge graph is a framework that integrates a large number of patient relevant datasets and complementary types of data modalities in a meaningful way. Machine learning and AI applications can then query this data to uncover previously unknown patterns and make novel target predictions. AI tools then enhance target assessment by surfacing the most relevant data to help scientist make data-driven decisions over which targets to prioritise. The information is stored in the knowledge graph in a scalable manner. 因此, 随着澳门第一赌城在线娱乐的数据和知识的增长和发展, 澳门第一赌城在线娱乐的图表也是如此, which means every newly designed experiment will benefit from everything learned before.
CKD包括许多不同的病因, 由多种途径和不同的潜在原因驱动, 不幸的是,它影响着全球十分之一的人. This complexity means it is challenging to understand the underlying drives of disease and identify novel targets. The aim, in the future, is to identify treatments that will halt or even reverse disease progression.
IPF的病因是特发性的或未知的. One of the challenges therefore in target discovery is knowing which biological mechanism to focus on. Being able to distinguish which mechanisms are driving a disease from those that are consequences of a disease can help to increase efficacy. Our aim is to treat the causative mechanisms of the disease as opposed to just the downstream symptoms so that we can prevent the progression of the disease.
The collaboration has already yielded a validated AI-generated target for CKD that has entered the AstraZeneca portfolio. For IPF we’ve identified potential targets and are currently experimentally validating them using novel experimental procedures like CRISPR.
The work we’ve developed together has furthered our understanding of these diseases. 知识图谱的使用, AI tools and machine learning models have put the spotlight on both well-established biology as well as more novel mechanisms. AI is no longer just a promise in drug discovery; we are transforming how new medicines are discovered.